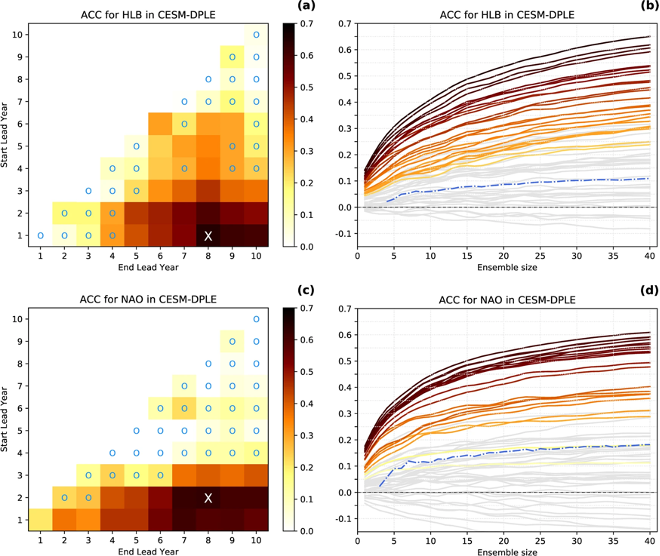
Figure 1: The predictive skill for the CESM-DPLE ensemble-mean measured by the anomaly correlation coefficient (ACC) for high-latitude blocking (HLB) in a and the North Atlantic Oscillation (NAO) in c. Each cell below the diagonal corresponds to a different lead-year range defined by the start and end lead-years. The cyan markers (o) indicate non-statistically significant correlations. In a and c, an X marker indicates the lead-year range with the highest ACC (0.65 for HLB and 0.63 for NAO). In b and d, the respective skill is computed as a function of the ensemble size (averaged for all possible member combinations). Each line corresponds to a different lead-year range. Lines in color correspond to statistically significant correlations for the full ensemble (N = 40) following the same color code as in a and b. The dashed-dotted lines show the skill of the sub-ensemble mean against a single member of the ensemble (averaged for all possible combinations). Reproduced from Athanasiadis et al. (2020).
Decadal predictions (those running up to 10 years into the future) have greatly improved in the last decade thanks to worldwide coordinated efforts (such as WCRP-DCPP). These led to model improvements, advancements in initialization strategies (beginning the model simulation using observed data), and significant increases in the sizes of model ensembles. Thus, decadal predictions are now run operationally across the world (see WMO World Lead Centre) allowing the development of skilful decadal climate services (e.g. see C3S_34c) to aid socio-economic planning and adaptation. This welcome progress calls for further improvements to make decadal predictions even more skilful and capable of providing reliable climate information at the local scale, including predictions on climate extremes and user-oriented indices.
To achieve this goal, EUCP has shown that specific objectives must be met. Similar to seasonal forecasts, decadal predictions suffer from low signal-to-noise ratios, meaning that the respective climate models under-represent the predictable components of climate variability. This can lead to the climate change signal being overwhelmed by natural variability in the predictions. This deficiency is particularly relevant to predicting climate extremes and variability in the Euro-Atlantic sector. Another key problem to be tackled is the still-significant model biases, which not only affect the all-important climate variability patterns and their teleconnections across the world, but also impact climate dynamics after initialization in relation to the so-called model drift. We highlight below three possible avenues for improving decadal predictions.
Increasing the ensemble size of individual model systems, as well as using multi-system ensembles, has been shown to increase the predictive skill for various climatic indices and variables in most parts of the world. As an example, Figure 1 shows how the anomaly correlation coefficient (ACC) for the wintertime North Atlantic Oscillation and high-latitude blocking in the North Atlantic increases monotonically with the ensemble size of the Community Earth System Model – Decadal Prediction Large Ensemble (CESM-DPLE). Clearly, the skill is still far from saturating at ensemble size N=40.
Such skill increases with the ensemble size occur thanks to noise cancellation accomplished via the ensemble averaging, which is more effective for larger ensemble sizes. The predictable component of the variability, in principle common to all members, survives the averaging, thus leading to higher skill.
On the other hand, recent studies have shown that increasing model resolution from about 1° (typical resolution of current decadal prediction systems) to 0.25° in the ocean has a significant positive impact on climatological biases across different HighResMIP models. This higher resolution allows the model to simulate much smaller-scale features. For example, Fig. 2 shows the respective Sea Surface Temperature bias reduction for a multi-model ensemble along with the associated bias reduction in European blocking, which is shown to be dynamically linked to the former. Such improvements in climatological model biases with increasing horizontal resolution are also expected to occur in decadal prediction systems (Roberts et al., 2020). Moreover, such increases in model resolution have been shown to bring improvements in mid-latitude air-sea interaction with a positive impact on the representation of climate variability (Haarsma et al., 2019; Bellucci et al., 2021; Tsartsali et al., in review) and near-term climate-change signal (Moreno-Chamarro et al., 2021).
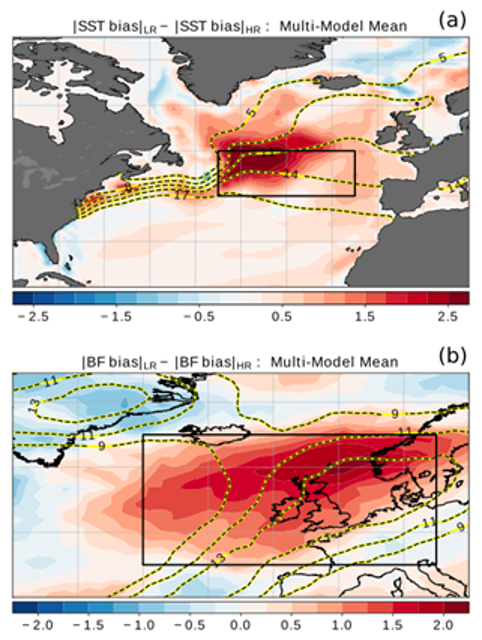
Figure 2: Differences in wintertime absolute bias between low-resolution (LR) and high-resolution (HR) multi-model means. (a) SST biases (shading, in K) with the HadISST2 1950–2014 climatology in contours (°C). (b) blocking frequency biases (shading, in % of blocked days) with the ERA-JOINT climatological blocking frequency in contours (% of blocked days). Adopted from Athanasiadis et al. (in review).
Beyond the discussed impacts of increasing the ensemble size (improving the predictive skill) and the horizontal model resolution (improving model realism and variability), there are a number of other recommendations for the next generation of decadal prediction systems. Among these, the realistic initialization of all climatic components (ocean, atmosphere, sea-ice and soil moisture) in a dynamically consistent way – via the use of coupled reanalyses – is expected to reduce initialization shocks in the models. Such developments would help the adoption of a seamless prediction strategy.
References in review:
Athanasiadis, P., Ogawa, F., Omrani, N.-E. et al. (in review) Mitigating climate biases in the mid-latitude North Atlantic by increasing model resolution: SST gradients and their relation to blocking and the jet. Journal of Climate
Tsartsali, E., Haarsma, R., de Vries, H. et al. (in review) Impact of resolution on atmosphere-ocean VMM and PAM coupling along the Gulf stream in global high resolution models. Climate Dynamics